Dr. Kuldeep Purohit
I am an AI/ML Researcher at Google, where my responsibilities entail the design and development of deep learning models tailored for edge computing, specifically targeting perception and scene understanding tasks. Prior to my tenure at Google, I served as an AI Research Scientist at Phiar, where I focused on designing and implementing deep learning models for Augmented Reality-based Autonomous Navigation. Until September 2021, I was a Post-doctoral Research Associate at the Computer Science and Engineering Department at Michigan State University with Prof. Vishnu Boddeti with research focused on on topics related to Computer Vision and Image Processing . I did my PhD(+MS) at Image Processing and Computer Vision Lab, Indian Institute of Technology Madras, Chennai under the supervision of Prof. A. N. Rajagopalan. I received my Bachelors Degree in Electrical Engineering from Indian Institute of Technology Mandi, Himachal Pradesh. My research lies at the intersection of Image Processing/Computer Vision and Deep Learning. My recent research projects focused on restoration of images and videos suffering from blur, low-resolution, rain, and haze and their utilization for scene segmentation and estimation of 3D geometry and motion. Email: kuldeeppurohit3@gmail.com
Google Scholar /
LinkedIn /
csauthors,net Profile /
CV
|
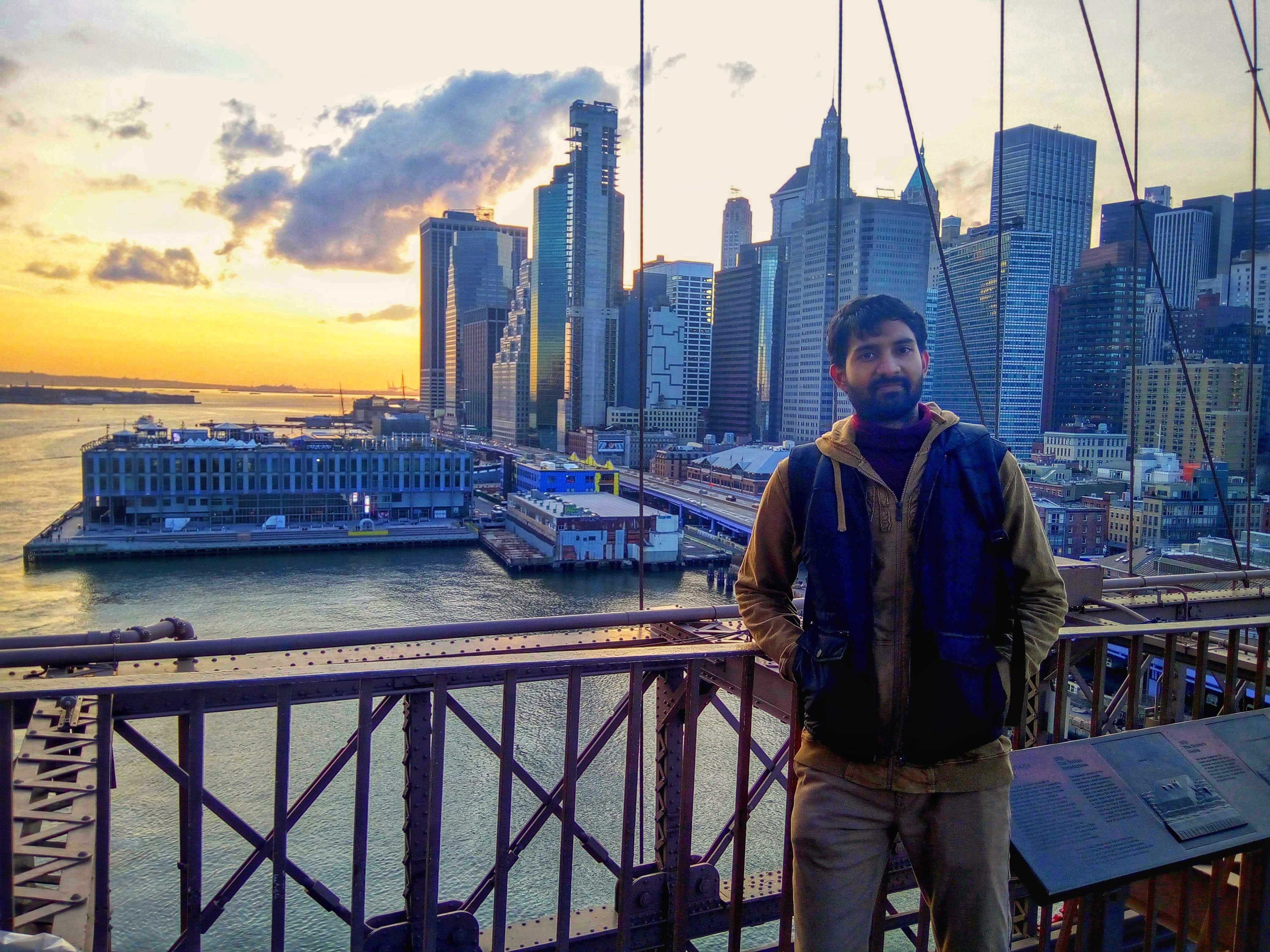 |
News
[2024.07] Invited to be an Area Chair for IEEE/CVF WACV 2025.
[2024.05] Invited to be a reviewer for NeurIPS 2024.
[2022.12] Joined Google (Mountain View, California) as an AI/ML Engineer.
[2022.02] Paper titled "Multi-planar geometry and latent image recovery from a single motion-blurred image" has been accepted at Machine Vision and Applications Journal.
[2021.10] Joined Phiar (California) as an AI Research Scientist.
[2021.07] Paper titled "Spatially-Adaptive Image Restoration using Distortion-Guided Networks" has been accepted at ICCV 2021 (IEEE/CVF International Conference on Computer Vision).
[2021.07] Paper titled "Distillation-Guided Image Inpainting" has been accepted at ICCV 2021 (IEEE/CVF International Conference on Computer Vision).
[2021.06] Invited Talk at the National Institute of Science Education and Research (NISER) Bhubaneshwar.
[2021.05] Invited lecture as a part of Computer Vision Talk series conducted by SciTech Talks.
[2021.03] Invited to be a reviewer for the IEEE Transactions on Pattern Analysis and Machine Intelligence (TPAMI).
[2020.06] Joined as a Post-doctoral Research Associate at the Computer Science and Engineering Department at Michigan State University under Prof. Vishnu Boddeti.
[2020.03] Our paper has been accepted at CVPR 2020, Seattle, USA.
[2020.01] I have been awarded ACM-India Travel Grant and AAAI Scholarship to present our paper at AAAI 2020, New York, USA.
[2019.12] Invited to be a reviewer for the IEEE Transactions on Image processing (TIP), IEEE Transactions on Multimedia (TMM) and International Journal of Computer Vision (IJCV) (Springer).
[2019.12] Invited to present my research at the Vision India Track in the National Conference on Computer Vision, Pattern Recognition, Image Processing and Graphics (NCVPRIPG 2019) (Springer).
[2019.11] Our team won the runner up prizes in ICCV-AIM 2019 Bokeh Effect Challenge and Realistic Image Super-resolution Challenge.
[2019.05] I have been awarded Google Travel Grant to present our paper at CVPR 2019, Long Beach, CA, USA.
[2019.04] Our team has claimed 1st position in CVPR-NTIRE 2019 Image Colorization Challenge. We are also among the finalists in Video Deblurring, Video Superresolution, and Image Dehazing challenges of NTIRE 2019.
[2018.12] Our work is selected for the Best Paper Award (Runner Up) in ICVGIP 2018.
[2018.08] Our team is among the finalists in all the 3 tracks of ECCV-PIRM 2018 Perceptual Image Super-resolution Challenge.
[2017.09] Our team secured 13th rank among 4000 participants in the Hackerearth's Deep Learning based Object Classification Challenge.
[2017.01] Started working as a Research Intern at KLA-Tencor, Chennai.
[2016.09] I will be presenting three papers from our lab at ICIP 2016, Phoenix, AZ, USA.
[2016.03] Started working on a sponsored Research Project with NIOT, Ministry of Earth Sciences, Govt. of India.
|
Publications and Preprints
|
|
SPAIR: Spatially-Adaptive Image Restoration using Distortion-Guided Networks Kuldeep Purohit, Maitreya Suin, A.N. Rajagopalan, and Vishnu Naresh Boddeti
ICCV 2021
Project Page
SPAIR is an effective two-stage fully convolutional architecture to generate distortion-aware features for removing spatially varying/sparse image degradations. Comprised of a Mask-guided Sparse Convolution (SC), Spatial Feature Modulator (SFM) and a Region-Guided Sparse Non-Local Module (SNL), SPAIR addresses the issue of image-agnostic and spatially-invariant features in existing restoration networks, leading to improved performance on various challenging degradations, such as rainstreak, raindrop, motion blur, shadow etc.
|
|
Spatially-attentive patch-hierarchical network for adaptive motion deblurring Kuldeep Purohit*, Maitreya Suin*, and A.N. Rajagopalan
CVPR 2020
Project Page
This paper tackles the problem of motion deblurring of dynamic scenes. Although end-to-end fully convolutional designs have recently advanced the state-of-the-art in non-uniform motion deblurring, their performance-complexity trade-off is still sub-optimal. Existing approaches achieve a large receptive field by increasing the number of generic convolution layers and kernel-size, but this comesat the expense of of the increase in model size and inference speed. In this work, we propose an efficient pixel adaptive and feature attentive design for handling large blur variations across different spatial locations and process each test image adaptively. We also propose an effective content-aware global-local filtering module that significantly improves performance by considering not only global dependencies but also by dynamically exploiting neighboring pixel information. We use a patch-hierarchical attentive architecture composed of the above module that implicitly discovers the spatial variations in the blur present in the input image and in turn, performs local and global modulation of intermediate features. Extensive qualitative and quantitative comparisons with prior art on deblurring benchmarks demonstrate that our design offers significant improvements over the state-of-the-art in accuracy as well as speed.
|
|
Region-Adaptive Dense Network for Efficient Motion Debluring Kuldeep Purohit and A.N. Rajagopalan
AAAI 2020
ArXiv Version
In this paper, we address the problem of dynamic scene deblurring in the presence of motion blur. Restoration of images affected by severe blur necessitates a network design with a large receptive field, which existing networks attempt to achieve through simple increment in the number of generic convolution layers, kernel-size, or the scales at which the image is processed. However, these techniques ignore the non-uniform nature of blur, and they come at the expense of an increase in model size and inference time. We present a new architecture composed of region adaptive dense deformable modules that implicitly discover the spatially varying shifts responsible for non-uniform blur in the input image and learn to modulate the filters. This capability is complemented by a self-attentive module which captures non-local spatial relationships among the intermediate features and enhances the spatially-varying processing capability. We incorporate these modules into a densely connected encoder-decoder design which utilizes pre-trained Densenet filters to further improve the performance. Our network facilitates interpretable modeling of the spatially-varying deblurring process while dispensing with multi-scale processing and large filters entirely. Extensive comparisons with prior art on benchmark dynamic scene deblurring datasets clearly demonstrate the superiority of the proposed networks via significant improvements in accuracy and speed, enabling almost real-time deblurring.
|
|
Depth-guided Dense Dynamic Filtering Network for Bokeh Effect Rendering Kuldeep Purohit*, Maitreya Suin, Praveen Kandula, and A.N. Rajagopalan
AIM Workshop and Challenge, International Conference on Computer Vision (ICCV 2019), Seoul, South Korea, November 2019
Our work presented at the International Conference on Computer Vision (ICCV) - Advances in Image Manipulation (AIM) Workshop 2019. Our team was a runner-up in both the tracks of Bokeh Effect Challenge (http://www.vision.ee.ethz.ch/aim19/).
|
|
Bringing Alive Blurred Moments Kuldeep Purohit*, Anshul Shah, and A.N. Rajagopalan
Accepted for Oral Presentation at IEEE Conference on Computer Vision and Pattern Recognition (CVPR 2019), Long Beach, CA, USA, June 2019
ArXiv version /
Supplementary /
Project Page
Designed a deep convolutional architecture to extract a sharp video from a motion blurred
image. The first stage involves unsupervised training of a novel spatiotemporal network
for motion extraction from short video sequences. The above network is utilized for guided
training of a CNN which extracts the same motion embedding from a single blurred image.
The above networks are finally linked with our efficient deblurring network to generate the
sharp video. Our framework delivers state-of-the-art accuracy in single image deblurring
and video extraction while being faster and more compact.
|
|
Mixed-Dense Connection Networks for Image and Video Super-Resolution Kuldeep Purohit*, Srimanta Mandal, and A.N. Rajagopalan
Elsevier Neurocomputing (Special Issue on Deep Learning for Image Super-Resolution) 2019
Paper Link
Proposed a deep architecture for image and video super-resolution, which is built using efficient convolutional units we refer to as mixed-dense connection blocks, whose design combines the strengths of both residual and dense connection strategies, while overcoming their limitations. We enable efficient super-resolution for higher scale-factors through our scale-recurrent framework which reutilizes the filters learnt for lower scale factors recursively for higher factors. We analyze the effects of loss configurations and demonstrate their utility in enhancing complementary image qualities. The proposed networks lead to state-of-the-art results on image and video super-resolution benchmarks.
|
|
Planar Geometry and Latest Scene Recovery from a Single Motion Blurred Image Kuldeep Purohit, Subeesh Vasu, M. Purnachandra Rao, and A.N. Rajagopalan
Under Review*
ArXiv Version
Existing works on motion deblurring either ignore the effects of depth-dependent blur or work with the assumption of a
multi-layered scene wherein each layer is modeled in the form of fronto-parallel plane. In this work, we consider
the case of 3D scenes with piecewise planar structure i.e., a scene that can be modeled as a combination of multiple
planes with arbitrary orientations. We first propose an approach for estimation of normal of a planar scene from a
single motion blurred observation. We then develop an algorithm for automatic recovery of a number of planes, the
parameters corresponding to each plane, and camera motion from a single motion blurred image of a multiplanar 3D scene.
Finally, we propose a first-of-its-kind approach to recover the planar geometry and latent image of the scene by
adopting an alternating minimization framework built on our findings. Experiments on synthetic and real data reveal
that our proposed method achieves state-of-the-art results.
|
|
Multi-level Weighted Enhancment for Underwater Image Dehazing Kuldeep Purohit, Srimanta Mandal and A.N. Rajagopalan
Journal of the Optical Society of America A (JOSA-A) *
Paper Link
Attenuation and scattering of light are responsible for haziness in images of underwater
scenes. We propose an approach to reduce this effect, based on the underlying principle is that enhancement at different
levels of detail can undo the degradation caused by underwater haze. The depth information is
captured implicitly while going through different levels of details due to depth-variant nature of
haze. Hence, we judiciously assign weights to different levels of image details and reveal that
their linear combination along with the coarsest information can successfully restore the image.
Results demonstrate the efficacy of our approach as compared to state-of-the-art underwater
dehazing methods.
|
|
EFFICIENT MOTION DEBLURRING WITH FEATURE TRANSFORMATION AND SPATIAL ATTENTION Kuldeep Purohit and A.N. Rajagopalan
Accepted at the IEEE International Conference on Image Processing (ICIP) 2019*
ArXiv Version
A preliminary version of our work "Spatially-Adaptive Residual Networks for Efficient Image and Video Deblurring"
|
|
Scale-Recurrent Multi-residual Dense Network for Image Super-Resolution Kuldeep Purohit*, Srimanta Mandal, and A.N. Rajagopalan
PIRM Workshop and Challenge, Eurpean Conference on Computer Vision Workshops (ECCVW 2018), Munich, Germany, September 2018
Paper /
Poster
A preliminary version of our Neurocomputing work, presented at the European Conference on Computer Vision (ECCV) - Perceptual Image Restoration and Manipulation (PIRM) Workshop 2018. Our team REC-SR was a finalist in all three regions of the Super Resolution Challenge (https://www.pirm2018.org/PIRM-SR.html).
|
|
Learning based Blur Detection and Segmentation
Kuldeep Purohit, Anshul B. Shah, and A.N. Rajagopalan
IEEE International Conference on Image Processing (ICIP 2018), Athens, Greece, October 2018
Paper Link /
Supplementary /
Poster
We present a robust two-level architecture for blur-based segmentation of a single image. First network is a fully convolutional encoder-decoder for estimating a semantically meaningful blur map from the full-resolution blurred image. Second network is a CNN-based classifier for obtaining local (patch-level) blur-probabilities. Fusion of the two network outputs enables accurate blur-segmentation using Graph-cut optimization over the obtained probabilities. We also show its applications in blur magnification and matting.
|
|
Color Image Super Resolution in Real Noise
Srimanta Mandal, Kuldeep Purohit, and A.N. Rajagopalan
Accepted for Oral Presentation at ACM Indian Conference on Computer Vision, Graphics and Image Processing (ICVGIP 2018), IIIT Hyderabad, India, december 2018
Accepted Version
Proposed an approach to super-resolve noisy color images by considering the color channels
jointly. Implicit low-rank structure of visual data is enforced via nuclear norm minimization
in association with color channel-dependent weights, which are added as a regularization
term to the cost function. Additionally, multi-scale details of the image are added to the
model through another regularization term that involves projection onto PCA basis, which
is constructed using similar patches extracted across different scales of the input image. Selected for the Best Paper Award (Runner Up) *: https://cvit.iiit.ac.in/icvgip18/bestpaperaward.php.
|
|
Mosaicing Deep Underwater Imagery Kuldeep Purohit,Subeesh Vasu, A.N. Rajagopalan, V Bala Naga Jyothi, and Ramesh Raju
ACM Indian Conference on Computer Vision, Graphics and Image Processing (ICVGIP 2016), IIT Guwahati, India, december 2016
main paper /
Supplementary /
Poster
This work deals with the problem of mosaicing deep underwater images (captured by Remotely Operated Vehicles), which suffer from haze, color-cast, and non-uniform illumination. We propose a framework that restores these images in accordance with a suitably derived degradation model. Furthermore, our scheme harnesses the scene-depth information present in the haze for non-rigid registration of the images before blending to construct a mosaic that is free from artifacts such as local blurring, ghosting, and visible seams.
|
|
Splicing Localization in Motion Blurred 3D scenes Kuldeep Purohit and A.N. Rajagopalan
IEEE International Conference on Image Processing (ICIP 2016), Phoenix, Arizona, September 2016
Paper /
Supplementary /
Poster
This work proposes an efficient algorithm for depth based segmentation using spatially-distributed blur-kernels present in a single motion-blurred image of a 3D scene. The segmentation is then further utilized to estimate global camera motion from a single blurred image of a 3D scene. Finally, local blur profiles are compared with the global motion model to highlight inconsistencies and detect spliced regions.
|
Co-authored Workshop Proceedings
|
|
NTIRE 2019 Challenge on Image Colorization: Report
Shuhang Gu, Radu Timofte, Richard Zhang, Maitreya Suin, Kuldeep Purohit , A. N. Rajagopalan, Athi Narayanan S., Jameer Babu Pinjari, Zhiwei Xiong, Zhan Shi, Chang Chen, Dong Liu, Manoj Sharma, Megh Makwana, Anuj Badhwar, Ajay Pratap Singh, Avinash Upadhyay, Akkshita Trivedi, Anil Saini, Santanu Chaudhury, Prasen Kumar Sharma, Priyankar Jain, Arijit Sur, Gokhan Ozbulak
IEEE Conference on Computer Vision and Pattern Recognition (CVPR) Workshop
PDF (Openacess)
Report describing various solutions to the Challenge on Image Colorization, NTIRE 2019
|
|
NTIRE 2019 Challenge on Video Deblurring: Methods and Results
Nah, Seungjun and Timofte, Radu and Baik, Sungyong and Hong, Seokil and Moon, Gyeongsik and Son, Sanghyun and Lee, Kyoung Mu and Wang, Xintao and Chan, Kelvin C.K. and Yu, Ke and Dong, Chao and Loy, Chen Change and Fan, Yuchen and Yu, Jiahui and Liu, Ding and Huang, Thomas S. and Sim, Hyeonjun and Kim, Munchurl and Park, Dongwon and Kim, Jisoo and Chun, Se Young and Haris, Muhammad and Shakhnarovich, Greg and Ukita, Norimichi and Zamir, Syed Waqas and Arora, Aditya and Khan, Salman and Khan, Fahad Shahbaz and Shao, Ling and Gupta, Rahul Kumar and Chudasama, Vishal and Patel, Heena and Upla, Kishor and Fan, Hongfei and Li, Guo and Zhang, Yumei and Li, Xiang and Zhang, Wenjie and He, Qingwen and Purohit, Kuldeep and Rajagopalan, A. N. and Kim, Jeonghun and Tofighi, Mohammad and Guo, Tiantong and Monga, Vishal
IEEE Conference on Computer Vision and Pattern Recognition (CVPR) Workshop
PDF (Openacess)
Report describing various solutions to the Challenge on Video Deblurring, NTIRE 2019
|
|
NTIRE 2019 Challenge on Video Super-Resolution: Methods and Results
Nah, Seungjun and Timofte, Radu and Gu, Shuhang and Baik, Sungyong and Hong, Seokil and Moon, Gyeongsik and Son, Sanghyun and Lee, Kyoung Mu and Wang, Xintao and Chan, Kelvin C.K. and Yu, Ke and Dong, Chao and Loy, Chen Change and Fan, Yuchen and Yu, Jiahui and Liu, Ding and Huang, Thomas S. and Liu, Xiao and Li, Chao and He, Dongliang and Ding, Yukang and Wen, Shilei and Porikli, Fatih and Kalarot, Ratheesh and Haris, Muhammad and Shakhnarovich, Greg and Ukita, Norimichi and Yi, Peng and Wang, Zhongyuan and Jiang, Kui and Jiang, Junjun and Ma, Jiayi and Dong, Hang and Zhang, Xinyi and Hu, Zhe and Kim, Kwanyoung and Kang, Dong Un and Chun, Se Young and Purohit, Kuldeep and Rajagopalan, A. N. and Tian, Yapeng and Zhang, Yulun and Fu, Yun and Xu, Chenliang and Tekalp, A. Murat and Yilmaz, M. Akin and Korkmaz, Cansu and Sharma, Manoj and Makwana, Megh and Badhwar, Anuj and Singh, Ajay Pratap and Upadhyay, Avinash and Mukhopadhyay, Rudrabha and Shukla, Ankit and Khanna, Dheeraj and Mandal, A.S. and Chaudhury, Santanu and Miao, Si and Zhu, Yongxin and Huo, Xiao
IEEE Conference on Computer Vision and Pattern Recognition (CVPR) Workshop
PDF (Openacess)
Report describing various solutions to the Challenge on Video Super-Resolution, NTIRE 2019
|
|
NTIRE 2019 Image Dehazing Challenge Report
Codruta O. Ancuti, Cosmin Ancuti, Radu Timofte, Luc Van Gool, Lei Zhang,Ming-Hsuan Yang, Tiantong Guo, Xuelu Li, Venkateswararao Cherukuri, Vishal Monga, Hao Jiang, Siyuan Yang, Yan Liu, Xiaochao Qu, Pengfei Wan, Dongwon Park, Se Young Chun, Ming Hong, Jinying Huang, Yizi Chen, Shuxin Chen, Bomin Wang, Pablo Navarrete Michelini, Hanwen Liu, Dan Zhu, Jing Liu, Sanchayan Santra, Ranjan Mondal , Bhabatosh Chanda, Peter Morales, Tzofi Klinghoffer, Le Manh Quan, Yong-Guk Kim, Xiao Liang, Runde Li, Jinshan Pan, Jinhui Tang, Kuldeep Purohit , Maitreya Suin, Raimondo Schettini, Simone Bianco, Flavio Piccoli, C. Cusano, Luigi Celona, Sunhee Hwang, Hyeran Byun, Subrahmanyam Murala, Akshay Dudhane, Harsh Aulakh, Zheng Tianxiang, Tao Zhang, Weining Qin, Runnan Zhou, Shanhu Wang, Jean-Philippe Tarel, Chuansheng Wang, Jiawei Wu
IEEE Conference on Computer Vision and Pattern Recognition (CVPR) Workshop
PDF (Openacess)
Report describing various solutions to the Challenge on Image Dehazing, NTIRE 2019
|
|
Enhancement and Photo-metric Stereo for SEM images Kuldeep Purohit, Arun M, Mahesh Mohan, and A.N. Rajagopalan
Project with KLA-Tencor, India
2017
Addressed the blind reconstruction problem in scanning electron microscope (SEM) photometric stereo for complicated semiconductor patterns to be measured.
Developed a scheme using domain-specific priors on surface and sensor patterns in the optimization framework for robust estimation of the 3D surface structures.
Also developed a user-centric detail enhancement scheme for improving visual quality of noisy SEM images.
Proposed appraoch was validated through experiments on real data and was deployed commercially.
|
|
Autonomous Bot for the Annual Intelligent Ground Vehicle Competition (IGVC)
Team Abhiyaan, Centre for Innovation (CFI), IIT Madras, India
2017
Report
Our team designed a fully autonomous all-terrain ground vehicle. I specifically worked on the Computer Vision Module which involved algorithm development for
real-time lane detection and obstacle segmentation task. The computer vision and path planning modules were integrated using ROS for autonomous navigation.
The design was used in Vehicles that represented IIT Madras in the Intelligent Ground Vehicle Competition (IGVC) in 2017 and 2018.
|
|
Multiple Target Detection and Tracking in Wide Area Surveillance Kuldeep Purohit and Arshad Jamal (Scientist E)
Project under Centre for Artificial Intelligence and Robotics, Defense Research and Development Organization, India
2012
Report
In this project, the problem of object detection and tracking in the challenging domain of wide area surveillance
has been tackled. This problem poses several challenges: large camera motion, strong parallax, large number of moving
objects, and small number of pixels on target, single channel data and low frame-rate of video. The method implemented
here overcomes these challenges when tested on UAV videos.
|
|